New study suggests that skill in modeling mountain rain and snow is bypassing the skill of observational networks
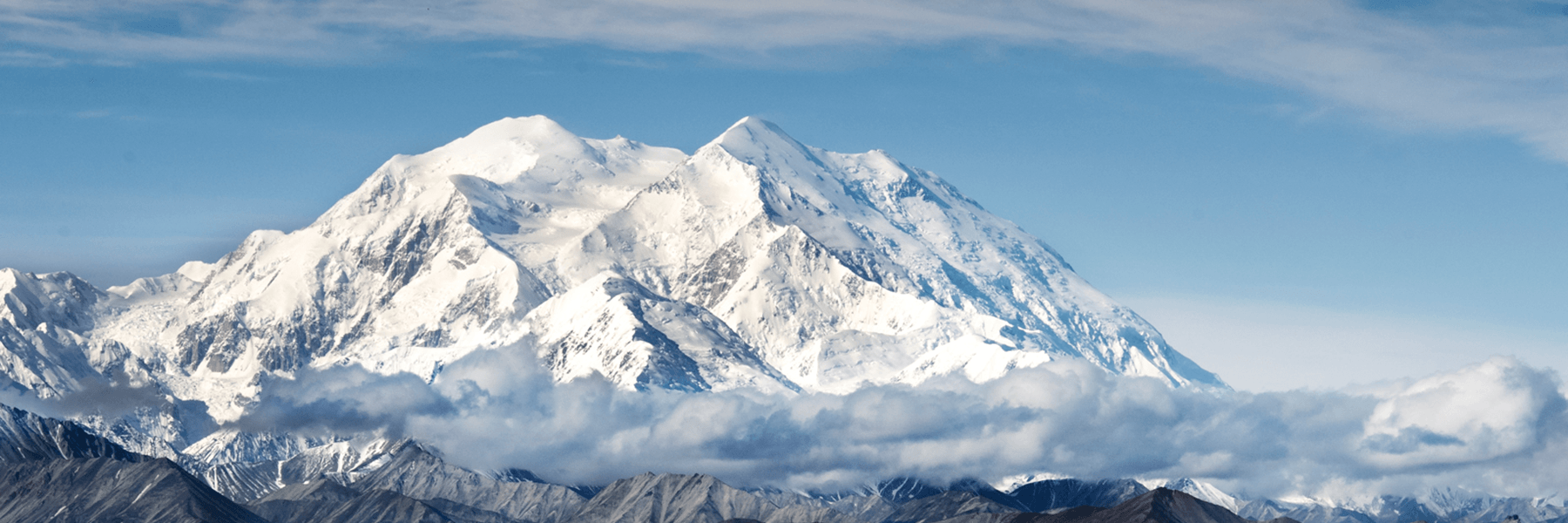
Rain and snow in the mountains, or the lack of it, can have major impacts downstream. Water managers, forecasters, and others need reliable information to make decisions, but observations in these regions are often sparse. A new study co-authored by a researcher from the ESRL Physical Sciences Laboratory evaluates the ability to observe and model rain and snow in mountainous, mid- and high-latitude areas. The article, to be published in the Bulletin of the American Meteorological Society, describes the limitations of precipitation observations in complex terrain, and how these limitations have been dealt with in the past, primarily using statistical methods to fill in between gauge observations of rain and snow. These observationally-based, gridded datasets have generally served as a benchmark for atmospheric models and as an input to hydrologic models. The study then reviews comparisons of gridded and atmospheric-model-based estimates of precipitation to independent data sources.
Comparisons of model estimates to independent rain and snow gauges reveal sometimes large errors in gridded precipitation estimates that ‘fill-in’ between gauges with statistical methods. Moreover, hydrologic model simulations of streamflow and snow are typically as close or closer to observations when forced by atmospheric models rather than gridded datasets. Precipitation estimates derived from streamflow observations also suggest statistically gridded datasets underestimate precipitation variability at high elevations. Taken collectively, this current research suggests that the ability to model total annual rain and snow in mountainous regions has reached or in some cases exceeded the accuracy of observationally-based, gridded estimates.
Mountain rain and snow estimates are critical for effective water resource management and flood prediction. This study suggests a necessary paradigm shift: No longer can gridded precipitation datasets serve as ‘gold standard’ benchmarks for atmospheric scientists and inputs for hydrologists. Instead, the study describes three ways forward: 1) investments in high-quality observations, including their quality control, 2) integration of various observations, and 3) closer collaborations between hydrologists and atmospheric scientists.
Authors of 'Our skill in modeling mountain rain and snow is bypassing the skill of our observational networks' are: Jessica Lundquist, Mimi Hughes, Ethan Gutmann, and Sarah Kapnick
Posted: September 4, 2019