Comparing precipitation forecasts to observation-based precipitation estimates to help evaluate and advance forecast models
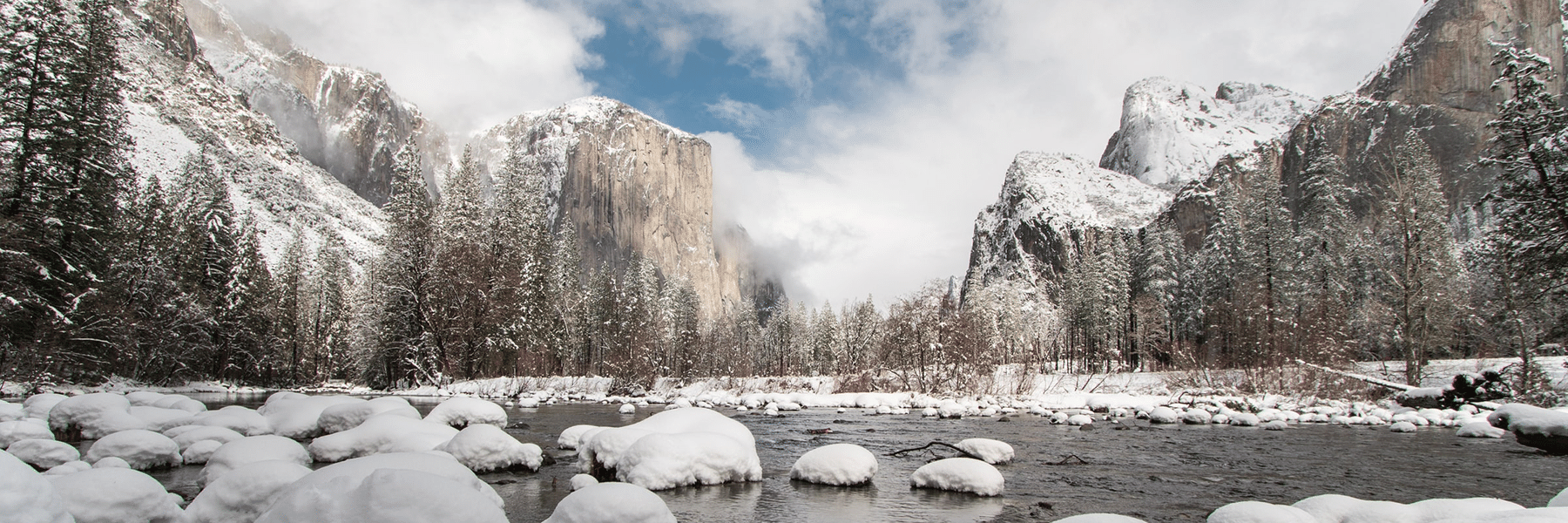
In Northern California, precipitation is difficult to measure at small location and time scales, in part because of the mountainous terrain and frequent changes between rain and snow. While many products exist that provide hourly precipitation estimates every few kilometers, each method of observation has strengths and weaknesses related to their ability to capture interactions between precipitation and the terrain, ability to install and maintain instruments, and ability to measure from space. This large uncertainty in estimated precipitation makes it difficult to evaluate the performance of high-resolution precipitation forecasts.
In a new study led by the Physical Sciences Laboratory, CIRES and NOAA researchers combined all available high-resolution precipitation estimates from gauges, radars, and satellites to provide a range of potential precipitation amounts—assuming that the correct value lies somewhere within the range of the estimates. They then compared the forecasted precipitation from NOAA’s HRRR model to the range of the estimates. Depending on how well the forecast precipitation amount agreed with the range of precipitation estimates from the various products, the researchers assigned a quality label of “good”, “possible”, “overestimate” or “underestimate” to the forecast to describe the model performance at a given location and time. Their findings were recently published in the journal Weather and Forecasting.
The method developed by the researchers classified precipitation forecasts at different time scales, either hourly, over an entire event, or over multiple cases, which helped to evaluate the model performance in a variety of ways. It was also used to evaluate two versions of the model, which helped track where forecasts get better or worse as the model is developed. In many cases, their method produced similar results to previous evaluations of the model, such as overestimating precipitation in the Sierra Nevada.
Forecast model evaluation is important for both the forecasters who use the model output to guide their forecasting, and for the developers who work to continually improve forecast models. For example, forecasters knowing that a particular model might typically underestimate rainfall in a given location means that when they deliver their forecasts to the public, they might predict slightly more rain than the model suggests. This is particularly important in heavy rain situations that might cause flooding or other hazards. Likewise, being able to quickly compare new model versions to the old helps model developers improve forecast accuracy.
J. Bytheway (PSL/CIRES), M. Hughes (PSL), R. Cifelli (PSL), K. Mahoney (PSL), J. M. English (GSL/CIRES), 2022: Demonstrating a Probabilistic Quantitative Precipitation Estimate for Evaluating Precipitation Forecasts in Complex Terrain. Wea. Forecasting, https://doi.org/10.1175/WAF-D-21-0074.1.
Posted: February 15, 2022