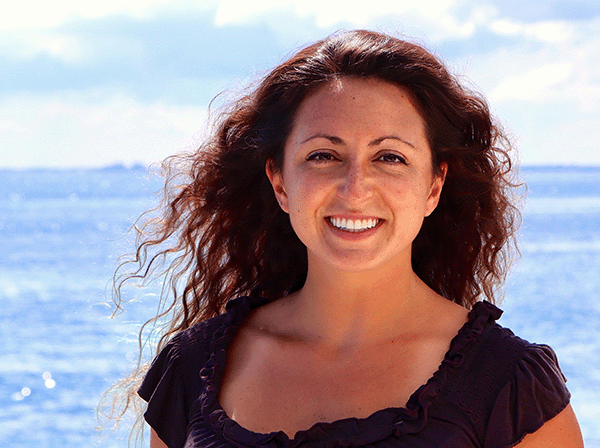
Identifying Subseasonal Forecasts of Opportunity Using Explainable AI
Marybeth Arcodia
Colorado State University
Tuesday, Apr 25, 2023, 2:00 pm
DSRC Room 2A305
JOIN THE WEBINAR:
GoTo MeetingAbstract
Extracting useful information about the chaotic Earth system enhances our ability to make accurate weather and climate predictions. In this seminar, I will discuss innovative approaches used to explore sources of predictability within the climate system that provide forecasts of opportunity – climate states that result in higher prediction skill– which ultimately lead to more confident climate forecasts from subseasonal (2 week-3 month) to decadal (5-10+ years) timescales. A machine learning approach using artificial neural networks is employed to investigate low frequency variability of subseasonal predictive skill, focusing on how the accuracy of forecasts of opportunity varies on decadal timescales. Further, eXplainable Artificial Intelligence (XAI) methods are used to understand the machine learning model’s decision-making strategy to identify sources of subseasonal predictability. This analysis is then extended to real-world data, indicating real and detectable signals in the climate system based on learned relationships from climate model data. Additionally, the influence of tropical modes of variability will be presented, particularly MJO and ENSO, on conditions in the United States. Constructive and destructive interference is shown to impact sea level heights, precipitation, and circulation and pressure patterns, potentially leading to extreme flooding or drought events. Localized impacts of climate change, specifically flooding and coastal sea level anomalies, will be presented, highlighting how our improved understanding of predictability translates to better preparation for climate extremes and long-term risk associated with global climate change.
SPEAKER BIO: Marybeth Arcodia currently works as a Postdoctoral Researcher for Colorado State University researching sources of predictability within the Earth's climate system on subseasonal to decadal timescales using explainable machine learning. Her work includes utilizing climate models and observational data to understand large-scale processes to identify and improve forecasts of opportunity. She received her PhD in Atmospheric Science from the University of Miami Rosenstiel School in 2021.
Seminar Contact: psl.seminars@noaa.gov