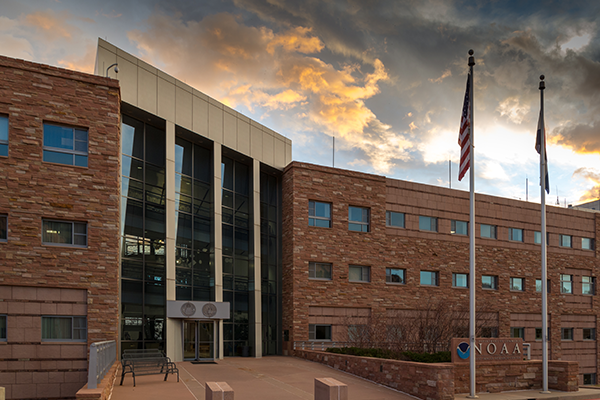
Projecting precipitation extremes in the warming climate
Aaron Wang
NOAA/PSL
Tuesday, Jun 20, 2023, 2:00 pm
DSRC Room 2A305
JOIN THE WEBINAR:
GoTo MeetingAbstract
A challenging issue in risk assessment industry is to project regional precipitation extremes in the warming climate. The resolution of the issue may be pursued by answering three questions: (a) To what extent are precipitation changes governed by a general increase of atmospheric humidity (“thermodynamic control”) or by changes in the statistics of mid tropospheric vertical velocity (“dynamic control”)? (b) What is the statistical distribution for precipitation? (c) How do we reproduce such distributions to predict the probability of extremes?
Despite popular belief that thermodynamic control is dominant in changing regional precipitation
patterns, it will be shown that changes in the daily mean precipitation, extreme (90 th percentile)
precipitation, and dry-day probability are much more consistent with changes in vertical velocity,
i.e. with dynamic control. The distribution of vertical velocity can be quantitatively understood
by adopting stochastically generated skewed (SGS) distribution theory, which has strong implication for extreme precipitation prediction.
In order to predict the probability of precipitation extremes, ensemble forecasting and stochastic parameterizations (SP) are suggested in the NWP model, where the SP is equivalent to the
correlated additive and multiplicative (CAM) noise that makes the PDF of vertical velocity
forecasts more realistically skewed and heavy-tailed. The SP also helps to reduce ensemble mean forecast errors and increase ensemble spread. A more realistic precipitation PDF is the key to probabilistic forecast and frequency analysis for extreme precipitation. An alternative way to efficiently generate probabilistic precipitation forecasts is being developed at Verisk. It adopts a framework that aims to debias coarse-scale GCM output and generate small-scale ensemble
forecasts by using machine learning. The rationale behind the framework could be attributed to
the principles of weather/climate prediction, especially the low predictability of the small scales
in the NWP models.
Seminar Contact: psl.seminars@noaa.gov