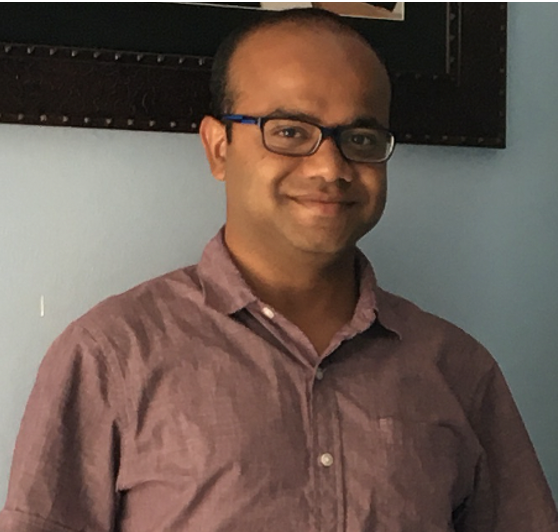
Process-Oriented Dynamical Models Evaluation for Seasonal Prediction through the Lens of Causal Network
Nachiketa Acharya
NOAA/PSL
Tuesday, Sep 12, 2023, 2:00 pm
DSRC Room 2A305
JOIN THE WEBINAR:
GoTo MeetingAbstract
In the realm of dynamical model diagnostics for seasonal forecasting, evaluating atmospheric dynamical interactions often entails measuring lagged correlations between distant climate variables. However, it's essential to note that correlation alone doesn't imply causation. Establishing cause-and-effect relationships requires more than correlation—it demands counterfactual dependence. Here, causal discovery algorithms excel, going beyond correlations to untangle common driver effects and indirect links.
This study introduces a process-oriented model evaluation framework using a causal network. This network, based on data-derived causal fingerprints, helps discern differences between models and observations. It achieves this by analyzing underlying physical processes that may contribute to model biases in simulating temperature and precipitation. Our approach involves three main components. First, we apply the innovative "DAG with No Tears" causal network algorithm to observed data, emphasizing reanalysis datasets. This unveils ocean-atmosphere interactions and their causality in regions influencing predictability. Then, a similar causal network is applied to atmosphere-ocean variables from dynamical models. Comparing causal fingerprints from observations and models involves pairwise assessments of all feasible links in the network. For quantification, we use the Matthews Correlation Coefficient (MCC) to capture differences between models and observations. This talk delves into the interpretability of this methodology, especially in the context of the Indian summer monsoon.
Seminar Contact: psl.seminars@noaa.gov