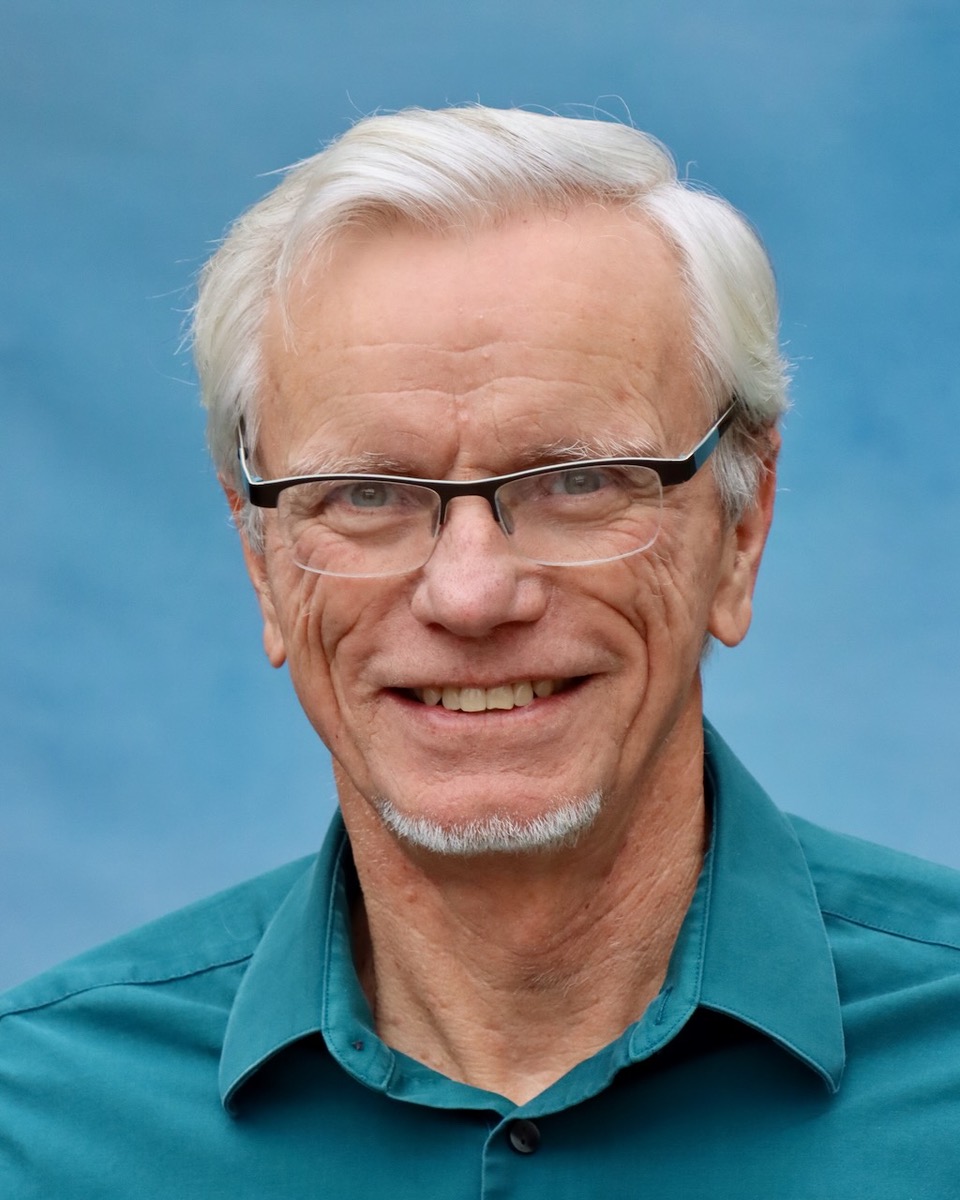
Toward Replacing Current NWP with Deep Learning Weather Prediction and Extensions to a Full Earth-System Model
Dale Durran
University of Washington
Tuesday, Oct 24, 2023, 2:00 pm
DSRC Room 2A305
Abstract
We compare the performance of a global deep-learning weather-prediction (DLWP) model with reanalysis data and forecasts from the European Center for Medium Range Weather Forecasts (ECMWF).
The model is trained using ECMWF ReAnalysis 5 (ERA5) data with convolutional neural networks (CNNs) on a HEALPix mesh using a loss function that minimizes forecast error over a single 24-hour period. The model predicts seven 2D shells of atmospheric data on an equal-area pixelization at resolutions of roughly 200 km
Notably, our model can be iterated forward indefinitely to produce forecasts at 3-hour temporal resolution for any lead time. We present case studies showing the extent to which the model is able to reproduce the dynamical evolution of atmospheric systems and its ability to learn “model physics” to forecast two-meter temperature and precipitation.
Extensions to a full earth-system model are presented using similar deep learning architecture to forecast sea surface temperatures. The SST model can be stably stepped forward for a year and shows skill in forecasting El Niños.
Seminar Contact: psl.seminars@noaa.gov