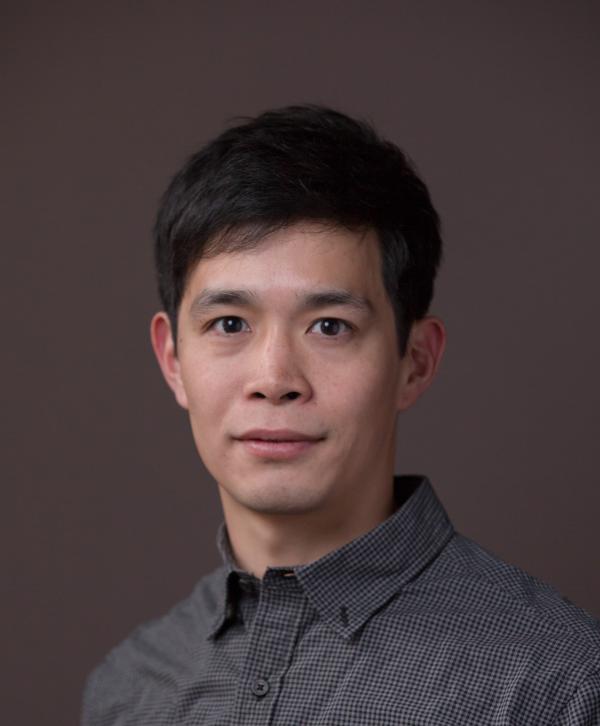
Fancy model analog for interpretable ENS forecasting
Kinya Toride
NOAA Physical Sciences Laboratory - CIRES CU Boulder
Tuesday, Mar 26, 2024, 2:00 pm MT
DSRC Room GC402

JOIN THE WEBINAR:
Google MeetAccess Code 718 983 414#
More phone numbers: https://tel.meet.com/tfh-jikn-jxu
Abstract
We introduce a hybrid method that integrates deep learning with model-analog for skillful and interpretable ENSO forecasting. This hybrid framework employs a convolutional neural network to estimate state-dependent weights to identify analog states. The advantage of our method lies in its physical interpretability, offering insights into initial-error-sensitive regions through estimated weights and the ability to trace the physically-based temporal evolution of the system through analog forecasting.
Results show a 10% improvement in forecasting sea surface temperature anomalies over the equatorial Pacific at a 12-month lead time with a perfect-model experiment. Furthermore, our hybrid model demonstrates improvements in boreal winter and spring initialization when evaluated against a reanalysis dataset. Our deep learning-based approach reveals state-dependent sensitivity linked to various physical processes, including the Pacific Meridional Modes, equatorial recharge oscillator, and westerly wind bursts. Notably, disparities emerge in the sensitivity associated with El Niño and La Niña events.
We find that sea surface temperature over the tropical Pacific plays a more crucial role in El Niño forecasting, while zonal wind stress over the same region exhibits greater significance in La Niña
prediction.
Bio: Kinya Toride received a B.Eng and M.Eng from the University of Tokyo, followed by an M.S. and Ph.D. from the University of California, Davis. He conducted postdoctoral research jointly at the University of Washington and the University of Tokyo, focusing on the subseasonal variability of atmospheric rivers and data assimilation of satellite-observed water vapor isotopes. In November 2022, he joined NOAA PSL and has since been working on developing new algorithms that leverage Al/ML to improve prediction and analyze the sources of hydroclimate variability.
Seminar Contact: psl.seminars@noaa.gov