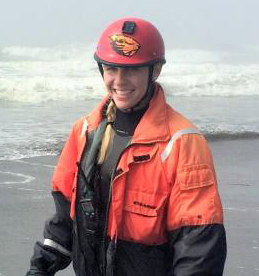
Using Stochastically Generated Skewed Distributions to Represent Hourly Nontidal Residual Water Levels at United States Tide Gauges
Paige Hovenga
NOAA Physical Sciences Laboratory
Tuesday, May 06, 2025, 2:00 pm MT
DSRC Room GC402

JOIN THE WEBINAR:
Google MeetAccess Code 718 983 414#
More phone numbers: https://meet.google.com/tel/tfh-jikn-jxu
Abstract
The daily likelihood of High Tide Flooding (HTF), currently predicted up to one year ahead by the National Oceanic and Atmospheric Administration (NOAA), can be expressed as the sum of a long-term trend, tide signal, and nontidal residuals (NTRs) whose probability density functions (PDFs) are assumed Gaussian. In this work, we show that hourly NTR distributions at 148 tide gauges along the U.S. coastline are better characterized with non-Gaussian (skewed, heavy tailed) ‘Stochastically Generated Skewed’ (SGS) distributions, resulting from a simple autoregressive model driven by correlated additive and multiplicative white noise (CAM noise), which could be related to physical processes. Most tide gauge
NTR observations exhibit some degree of non-Gaussian characteristics, especially along the U.S. Gulf and East Coasts, where the SGS distributions show large differences in likelihoods of high-water extremes compared to a Gaussian PDF, notably during summer. Conversely, along the U.S. West Coast, where
variability is often dominated by monthly time scales, many locations have nearly Gaussian PDFs, although SGS PDFs still better characterize winter conditions including an enhanced likelihood of high-water extremes. For evaluating high-water event probabilities, the SGS distribution is no more sensitive to limited observations than kernel density estimation and Generalized Extreme Value methods. Tail probabilities for all three methods are generally similar, despite the SGS approach fitting the observed time series rather than fitting the observed raw histogram, unlike the other methods. Our results may
improve NOAA’s HTF predictions, and more broadly, are relevant in developing inundation mitigation strategies for future sea level scenarios.
Bio: Paige Hovenga is a Research Physical Scientist in NOAA's Physical Sciences Laboratory. She earned a B.S. and M.S. in Civil Engineering from the University of Central Florida and a Ph.D. in Coastal and Ocean Engineering from Oregon State University where her research focused on coastal morphodynamics of
beaches and dunes. Her most recent work has been geared towards improving coastal water level estimates to improve predictions and projections of flooding.
Seminar Contact: psl.seminars@noaa.gov